- SIFT is a method to:
- Detect keypoints (interesting, stable points in the image)
- Describe them in a way that is invariant to scale and rotation
- Scale Invariance
- SIFT is robust to images taken at different zoom levels (scales).
- It does this by detecting keypoints in multiple scales, not just a single image resolution.
- Detector: Difference of Gaussian (DoG)
- L(x,y,σ) : the image blurred with a Gaussian filter of scale σ
- k : a constant factor for the next level of scale
- Subtracting two Gaussians of different scales creates the DoG image
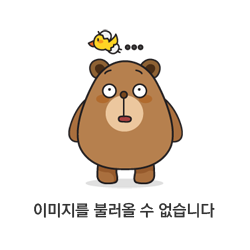
- Each row of rectangles represents images at different blur levels (σ) of the same input image.
- The bottom row is the first octave (original resolution)
- The rows above are next octaves (downsampled by 1/2 each time)
- Within each octave, the image is blurred incrementally using different values of Gaussian σ (standard deviation)
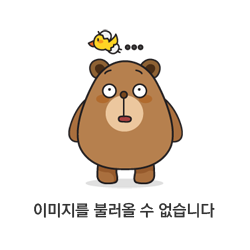
- Each oval between pairs of rectangles is computing the Difference of Gaussians:
- This subtracts two blurred images at slightly different levels of blur, resulting in DoG images.
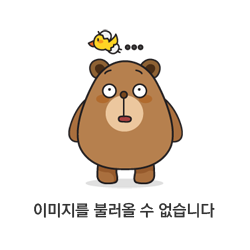
- Once a set of DoG images is computed for one resolution (octave), the image is downsampled by 2 (half size), and the process repeats:
- New blurred versions are created at that smaller size
- More DoG images are computed
- This helps SIFT detect features that may appear at different real-world sizes (scales).
Input image
↓
Apply Gaussian blur repeatedly → [Gaussian Pyramid]
↓
Subtract adjacent Gaussians → [DoG images]
↓
Find keypoints as local extrema in DoG stack
↓
Downsample and repeat for next octave
- Divide the Patch Around the Keypoint
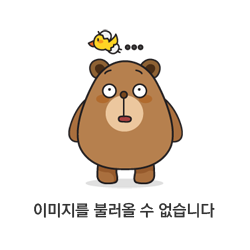
- Around each keypoint, take a 16×16 pixel window.
- Split it into 4×4 = 16 cells (each is 4×4 pixels).
- In each cell, compute a histogram of gradient orientations (8 bins).
- So each 4×4 block becomes an 8-element orientation histogram.
- Descriptor Size
- You have 16 blocks (4×4)
- Each block has 8 orientation values
- Resulting in:
16×8=128
- The whole descriptor is rotated so that it aligns with the keypoint’s dominant orientation
- This ensures that the same patch rotated in a different image will still match.
Feature | SIFT (Scale-Invariant Feature Transform) | SURF (Speeded Up Robust Features) | KAZE (Nonlinear Scale Space) |
Scale Space | Gaussian pyramid (linear scale space) | Gaussian pyramid (linear scale space) | Nonlinear diffusion (preserves edges and boundaries) |
Detector | Difference of Gaussian (DoG) | Hessian matrix (approximated by box filters) | Determinant of Hessian matrix in nonlinear space |
Descriptor | Gradient orientation histograms | Haar wavelet responses | First-order image derivatives |
Rotation Invariance | Yes | Yes | Yes |
Illumination Invariance | Normalized gradient magnitude | Normalized Haar responses | Normalized first-order derivatives |
Computation Speed | Slower | Faster than SIFT | Slower (due to nonlinear PDE solving) |
Robustness | Good for scale and rotation | Good, faster alternative to SIFT | Better at preserving edge structures and boundaries |
Descriptor Dimension | 128 | 64 | 64 |
Key Innovation | Scale-space extrema + gradient histograms | Integral image + box filters for speed | Nonlinear scale space via anisotropic diffusion |
https://blog.naver.com/pig9456/223476127316
'Autonomous Vehicle > Video Geometry' 카테고리의 다른 글
KAZE (0) | 2025.04.03 |
---|---|
SURF (Speeded Up Robust Features) (0) | 2025.04.03 |
Homography (0) | 2024.07.25 |
Camera Calibration (0) | 2024.07.23 |
3D Transformations (0) | 2024.07.19 |